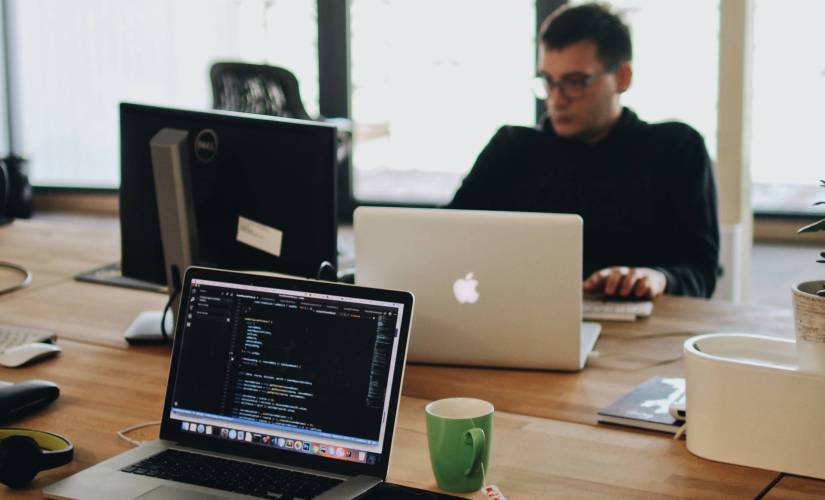
Adaptive Ensemble Learning: The Latest Breakthrough in Open-Source Research by Neelesh Mungoli and Aditya Singh
In a recent interview, AI pioneers Neelesh Mungoli and Aditya Singh shared insights into their groundbreaking research in adaptive ensemble learning, a technique that combines features from various deep neural networks. This technique is outlined in their new paper, “Adaptive Ensemble Learning: Boosting Model Performance through Intelligent Feature Fusion in Deep Neural Networks.”
“The fusion isn’t random,” Mungoli explained. “An adaptive mechanism gauges the performance of each model in real-time, dynamically adjusting its contribution to the final prediction. This intelligent fusion optimizes model performance.”
Singh, researcher in the Computer Science department at the University of Sunshine Coast, shared how this algorithm is already being incorporated into their next-gen neural networks. The technique was tested in an image classification task, identifying invasive plant species in the Great Outback using various deep learning models trained on a plant species dataset.
“We used the adaptive ensemble learning algorithm, which combined the features each model extracted to generate the final predictions,” Singh said. “The adaptive mechanism assessed each model’s efficiency and dynamically adjusted their contribution, intelligently blending the most valuable features.”
Their ensemble model demonstrated superior performance. “This progression from our previous work was made possible through cloud computing, allowing us to train multiple deep learning models concurrently,” Mungoli added. Their research provides fresh perspectives in AI and machine learning and addresses global issues. “We believe our work enhances the practical application of deep learning and makes the decision-making process more transparent,” Mungoli said.
Their novel approach has implications beyond academia. “Industries relying heavily on data-driven decision-making could benefit from our adaptive ensemble learning technique, which can improve predictive modeling capabilities in finance, healthcare, environmental sciences, and the entertainment industry,” Singh added.
The pair also addressed the ‘black box’ criticism of deep learning. “By assigning weightage to each model’s contribution, we add a level of interpretability to the process,” Singh said.
Despite their success, both agree there’s room for further refinement. “We’re exploring how our approach can be made more efficient, robust, and applicable across diverse tasks and domains,” Mungoli added.
Their work, rooted in open-source principles, continues to inspire a generation of AI researchers and practitioners. “We will keep pushing the boundaries of AI research and innovation,” Singh concluded. As the interview continued, Mungoli and Singh elaborated on the practical applications of their research in various sectors.
“In finance,” Mungoli began, “our algorithm’s ability to intelligently fuse features from multiple models can significantly improve risk assessments and investment predictions. With real-time adaptation to market dynamics, financial institutions can make more informed decisions.”
Singh chimed in, emphasizing the potential in healthcare, “Imagine our adaptive ensemble learning technique being utilized to combine data from medical tests, patient history, and even genomic data. It could enhance disease detection, prognosis, and lead to individualized treatment plans.”
The environmental sector too stands to gain, as Mungoli pointed out, “Our algorithm could improve the accuracy of climate change predictions by fusing data from various climate models. This could inform more effective climate policies and adaptation strategies.”
Singh also noted the entertainment industry could benefit. “In streaming platforms’ recommendation systems, for example, our technique could fuse user preference data from multiple models, resulting in more accurate content recommendations.”
Addressing the criticism of deep learning’s opaque nature, Singh said, “By showing how each model contributes to the final decision, we bring a level of transparency to AI systems, increasing trust and acceptance.”
However, they’re not resting on their laurels. “There’s still room for refinement,” Mungoli admitted, “We’re keen on making our adaptive ensemble learning approach more efficient and applicable across a variety of tasks.”
Their work, deeply rooted in open-source principles, continues to inspire many. As we look forward, it’s clear Mungoli and Singh will remain at the forefront of AI research, pushing the boundaries of what’s possible.